The importance of analyzing, monitoring, and inspecting food products during the packaging process is well-known within the agriculture and food packaging industries.
Companies involved with agricultural produce face issues such as contamination with stones or other debris, unsealed or broken packages, mislabeled products, and spoiled produce. It is important that solutions that address such problems are available using current technologies, such as InGaAs SWIR and CCD/CMOS.
Challenges in the Quality and Safety of Food Inspection
Cameras and sensors are included in the devices' design to improve inspection, sorting, and quality. These systems enable the identification of these issues in food products and packaging before shipping. Vision inspection can prevent low-quality or unsafe food from reaching shelves and reduce business expenses from returning defective products.
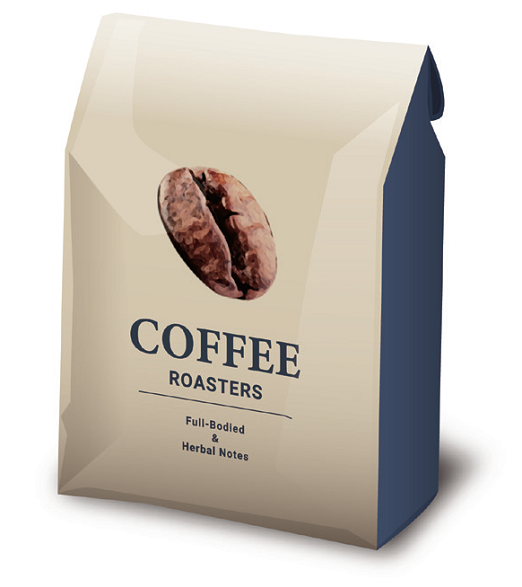
Image Credit: Hamamatsu Photonics Europe
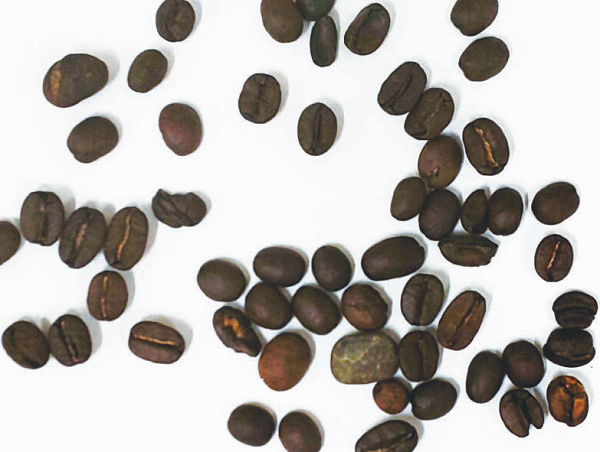
Difficulty detecting the stone among coffee beans with visible imaging. Image Credit: Hamamatsu Photonics Europe
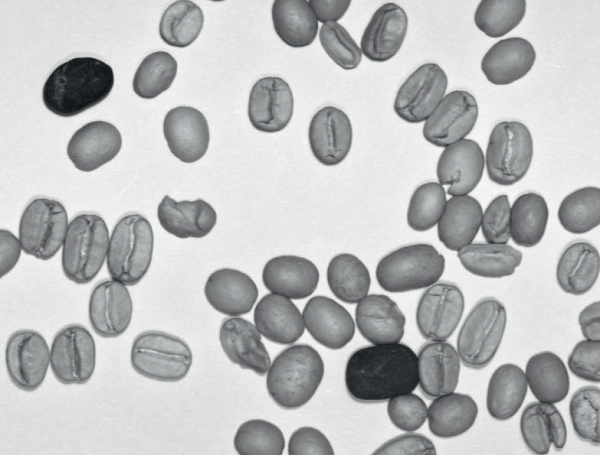
Clear identification of stone among coffee beans with C12741–03 InGaAs camera. Image Credit: Hamamatsu Photonics Europe
Coffee production is an example of a process that can benefit from machine vision. Coffee beans are dried on a tarp and subsequently raked up and put in a hopper.
During the raking process, rocks and other debris can easily become mixed with the coffee beans. These impurities must be removed before the packaging stage. If missed, the stones will be packaged with the coffee beans, presenting sanitary issues and causing coffee grinders to break.
Machine vision can be used to distinguish between objects which are similar in size and shape.
Placing cameras and detectors in strategic locations throughout the line will help identify materials based on their SWIR spectra, enabling distinction between coffee beans and stones.
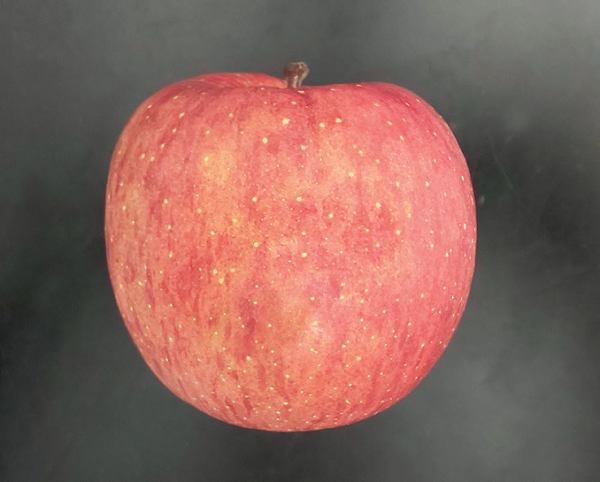
Visible wavelength image of an apple. Image Credit: Hamamatsu Photonics Europe
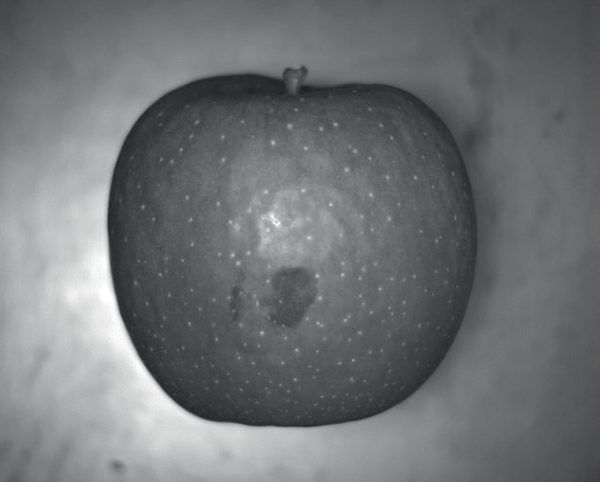
Infrared image of an apple, taken with C12741-03 InGaAs camera, shows hidden defects. Image Credit: Hamamatsu Photonics Europe
Moisture content significantly impacts the quality and shelf life of agricultural products. Small traces of additional moisture can indicate the start of mold. A bruise occurs when oxygen penetrates the skin or peel.
Bruised fruit or vegetables will have brown spots and may eventually turn brown entirely. Bruised fruit, while safe to eat in most cases, is not aesthetically appealing or can be over-ripe.
For example, apples traveling down the conveyor belt are scanned using CMOS and InGaAs cameras. The CMOS camera will show visible defects, whereas the InGaAs camera will show defects beginning to form under the skin that is undetectable to the human eye.
The food sorting inspection process is optimized using different high-speed imaging devices strategically positioned on the conveyor belt. These sensors will scan produce automatically, detecting visible and invisible defects on produce that should be filtered out, preventing them from reaching the store.
Sustainably Meeting Increasing Food Demands
With the world population expected to grow to 9.7 billion by 2025, agricultural companies face the challenge of keeping up with the increase in demand for high-quality food. They must continually optimize the food sorting and inspection process.
Human vision is unreliable as similar-looking colors can easily be confused and defects under food skin or packaging cannot be identified.
On the other hand, machine vision can reduce errors in food sorting and packaging by detecting invisible issues to the naked eye.
Moisture content on or beneath the skin of food is one such example, as well as capturing leaky packaging and differentiating between foreign objects and goods of similar colors and shapes.
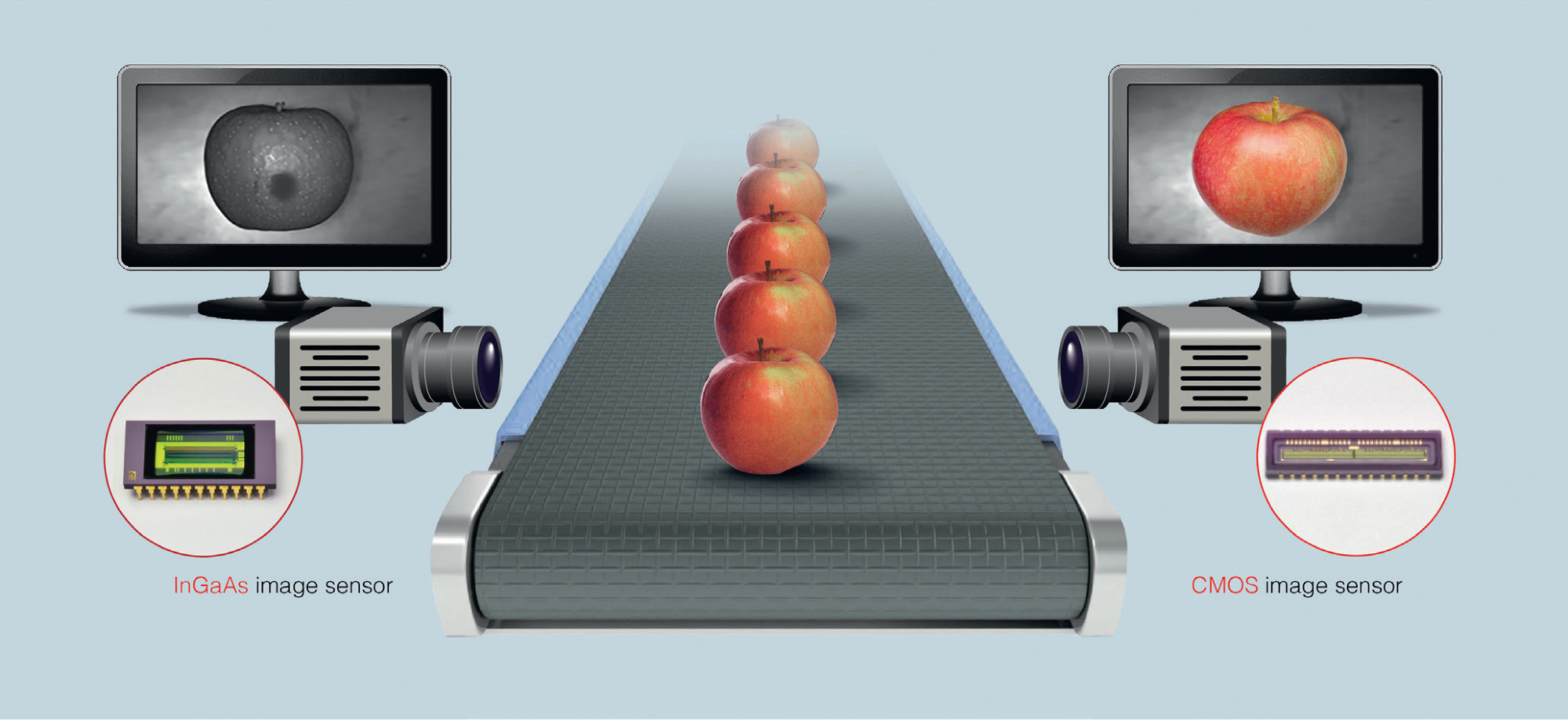
As apples travel down the conveyor belt, they are scanned using InGaAs and CMOS cameras. The InGaAs camera will show defects beginning to form under the skin that a human eye cannot see; the CMOS camera will show visible defects. Image Credit: Hamamatsu Photonics Europe
The Technological Solutions
Hamamatsu Photonics offers a broad selection of CMOS image sensors in linear and area arrays for UV-VIS imaging used in food inspection.
CMOS linear image sensors with high sensitivity from UV to the near-infrared region up to 1100 nm are available with a range of pixel configurations (from 128 pixels to 4096 pixels), line speeds, and sensitivity.
CMOS area image sensors are available in various array configurations in the range of 30 x 30 pixels to 1280 x 1024 pixels.
InGaAs linear image sensors are ideal for in-line sorting of agricultural products due to their high-speed line rate and high sensitivity. They are available in various cutoff wavelengths, line readout speeds, and pixel counts.
InGaAs area image sensors are suitable for hyperspectral imaging. These area arrays are characterized by high-speed frame rate, low readout noise, and high sensitivity. They are available in various cutoff wavelengths, pixel arrays, and frame rates.
InGaAs cameras provide plug-and-play solutions using 1D or 2D InGaAs image sensors. They are also an easy solution for multispectral/hyperspectral imaging (HSI) cameras to be built based on the original camera.
SWIR hyperspectral imaging is just beginning for food processing applications such as almond grading and quality inspection, capable of detecting foreign objects and grading food such as nuts or identifying ingredients.
Hamamatsu Photonics provides a complete product line with various cutoff wavelengths from 1.7 μm to 2.5 μm, also providing options from sensors to modules.
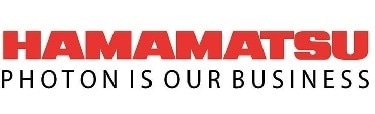
This information has been sourced, reviewed and adapted from materials provided by Hamamatsu Photonics Europe.
For more information on this source, please visit Hamamatsu Photonics Europe.