Adaptive filtering algorithms are a crucial part of systems that detect and exclude outliers such as travel time data acquired from Wi-Fi and Bluetooth MAC address detection. The primary challenge here is to design an algorithm with the capacity to capture abrupt changes in travel time patterns whilst working well in both arterial and highway scenarios.
This article sets forth data samples captured by SMATS’ sensors in numerous deployment cases and demonstrates how the algorithm designed for the task filters the outliers from the dataset. The SMATS algorithm has been developed and tested to work with both high and low sample sizes as well as arterial and freeway travel time data. The subsequent examples illustrate how this algorithm operates in varying situations.
Case 1: Highway Traffic, Incident
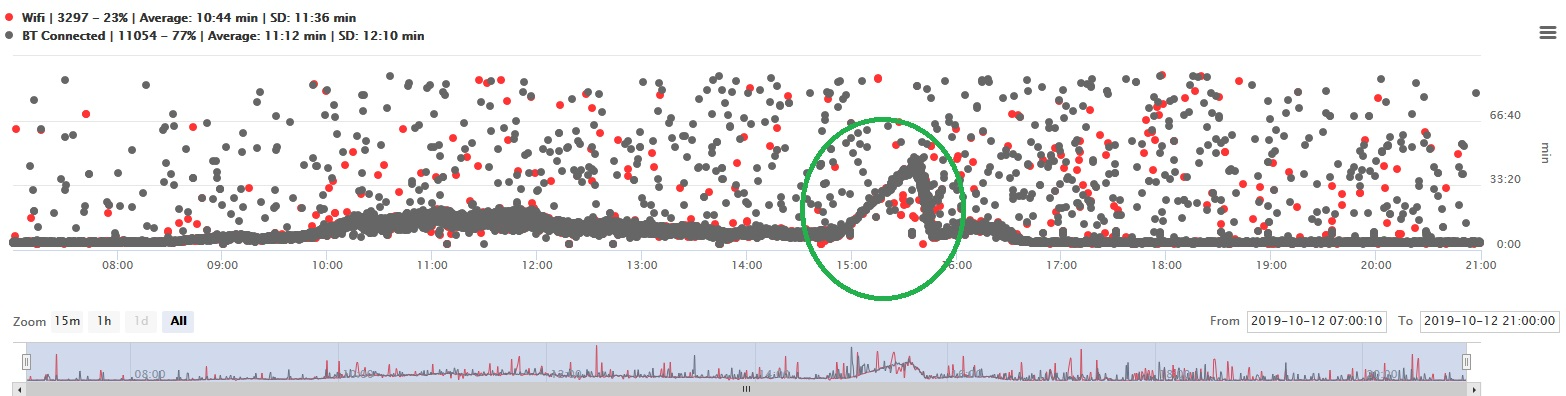
Figure 1. Matched travel time data, Incident, Highway. Image Credit: SMATS Traffic Solutions Inc.
Figure 1 exhibits one-day of raw travel time data for a busy highway in Toronto, Canada. As illustrated in Figure 1, the data is often noisy, with an increase in the travel time at around 15:00 due to a lane closure as the result of an accident. Once the accident has been cleared, at 15:45, a sharp reduction in the travel time occurs. Figure 2 exhibits how an Adaptive Filtering Algorithm can correctly filter the outliers and track sudden changes.
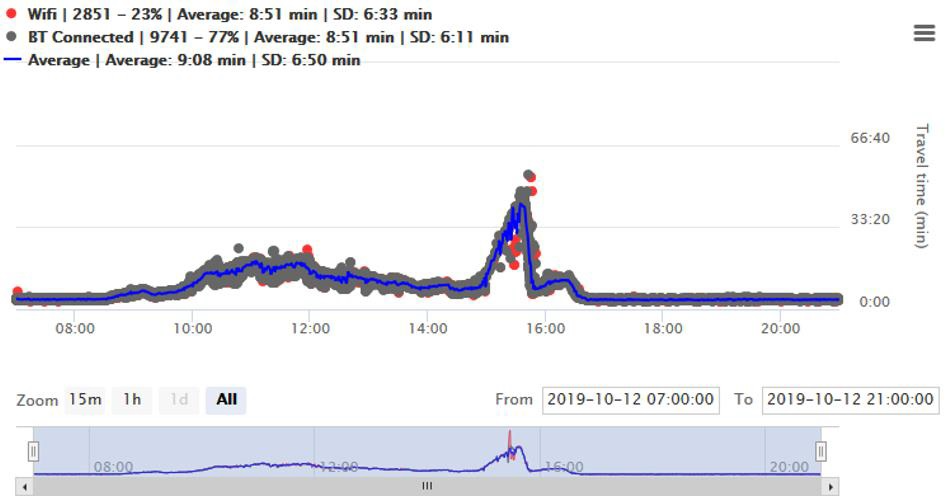
Figure 2. Filtered travel time data, Incident, Highway. Image Credit: SMATS Traffic Solutions Inc.
Case 2: Highway Traffic, Peak Hours
Figures 3 and 4 display another example from a highway, this time in Minneapolis, US. As illustrated, the SMATS’ filtering algorithm detected the peak evening rush hour on the highway and could properly follow the traffic pattern.
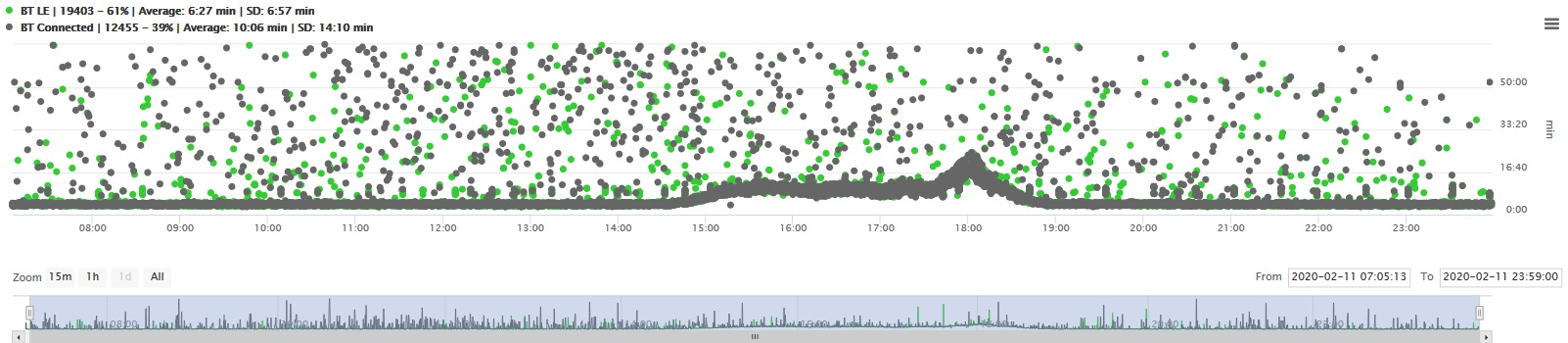
Figure 3. Matched travel time data, PM Peak, Highway. Image Credit: SMATS Traffic Solutions Inc.
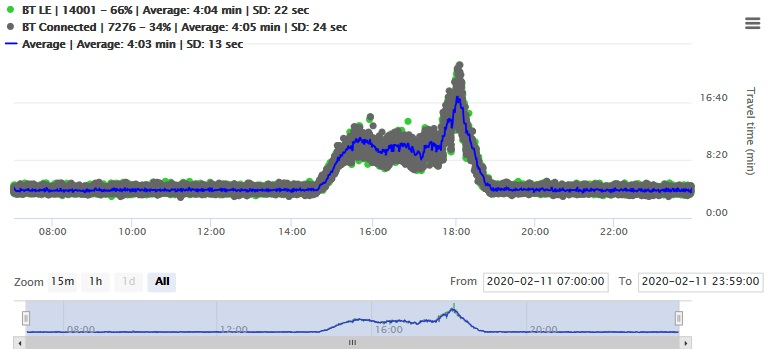
Figure 4. Filtered travel time data, PM Peak, Highway. Image Credit: SMATS Traffic Solutions Inc.
Case 3: Arterial Traffic with Signalized Intersection
An additional advantage of SMATS’ Adaptive Filtering Algorithm is that it can sufficiently operate for both freeways and signalized arterial roadways. Figures 5 and 6 respectively exhibit both raw and filtered travel time data of signalized arterial roadways. The matched data is somewhat scattered because of the presence of an intersection.
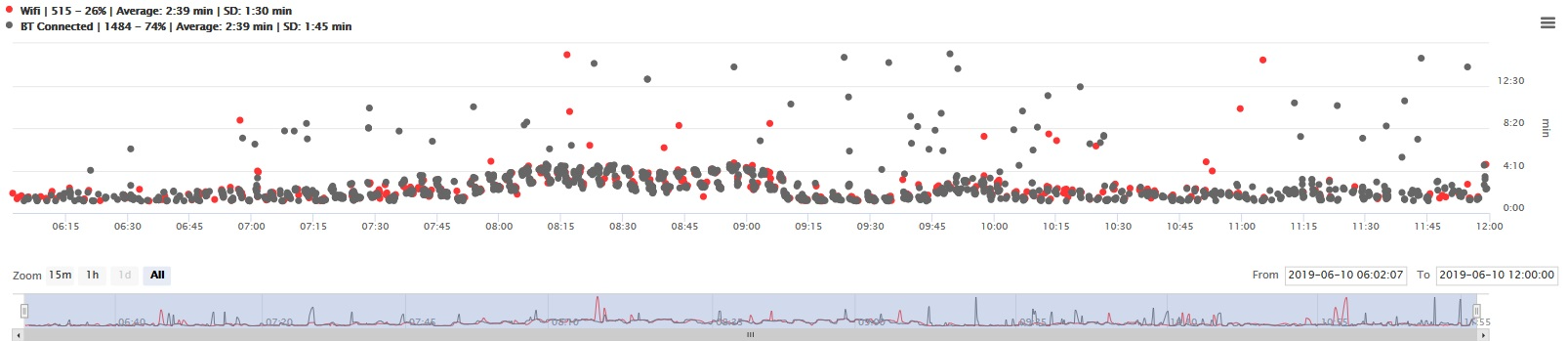
Figure 5. Matched travel time data, AM Peak, Signalized Intersection. Image Credit: SMATS Traffic Solutions Inc.
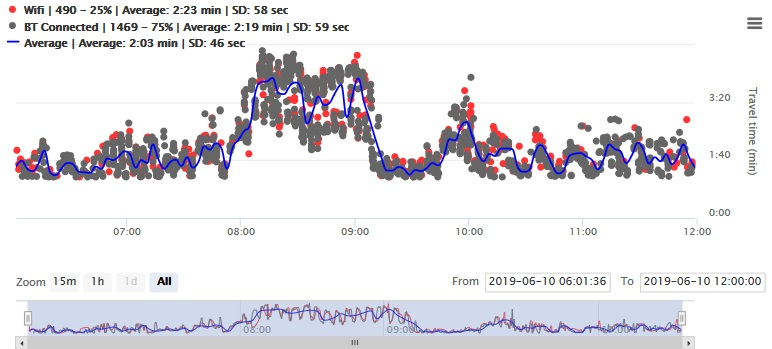
Figure 6. Filtered travel time data, AM Peak, Signalized Intersection. Image Credit: SMATS Traffic Solutions Inc.
Case 4: Faster Moving Traffic in Parallel with the Main Traffic
Figures 7 and 8 demonstrate a scenario in which there are two parallel traffic streams moving at varying speeds. One stream is dedicated for the use of general public traffic (fast) and the other is for heavy goods and truck traffic (slow) entering a Port in Los Angeles, US. As visible in Figure 7, the raw data incorporates the travel time data for both the fast and slow streams.
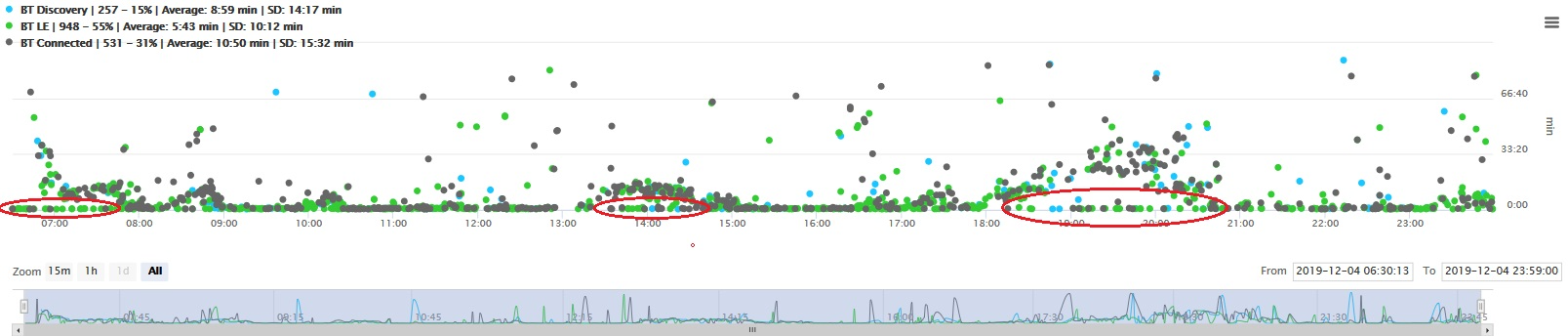
Figure 7. Matched travel time data, Parallel Faster Traffic, Port. Image Credit: SMATS Traffic Solutions Inc.
Therefore, the heavy good and truck traffic has been captured appropriately and the Port can monitor the entrance wait-time-data in real-time, after applying SMATS’ Adaptive Filtering Algorithm to the data. Figure 8 demonstrates the high-performance of the algorithm when it comes to detecting and removing the faster-traffic data from the overall dataset.
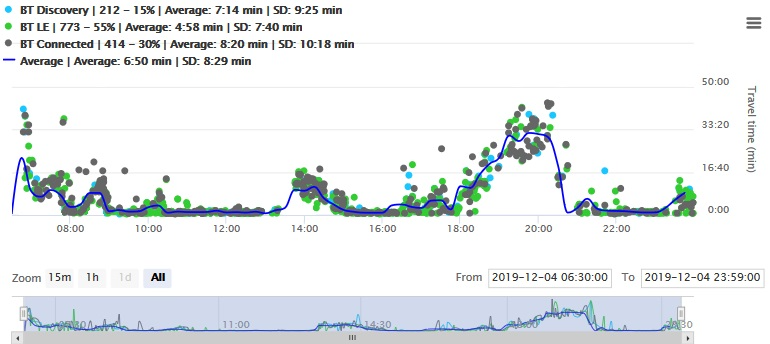
Figure 8. Filtered travel time data, Parallel Faster Traffic, Port. Image Credit: SMATS Traffic Solutions Inc.
Summary
SMATS’ Adaptive Filtering Algorithm has been tested across a range of scenarios based on varying datasets such as sudden changes in travel time due to incidences, low sample size, busy highways, arterial signalized intersections, truck traffic entering ports, etc. In all instances, SMATS’ Adaptive Filtering Algorithm can sufficiently identify outliers accurately and precisely represent the travel time trends.
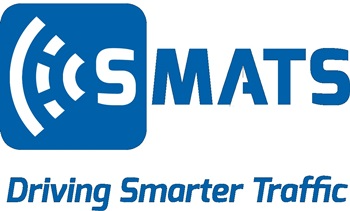
This information has been sourced, reviewed and adapted from materials provided by SMATS Traffic Solutions Inc.
For more information on this source, please visit SMATS Traffic Solutions Inc.