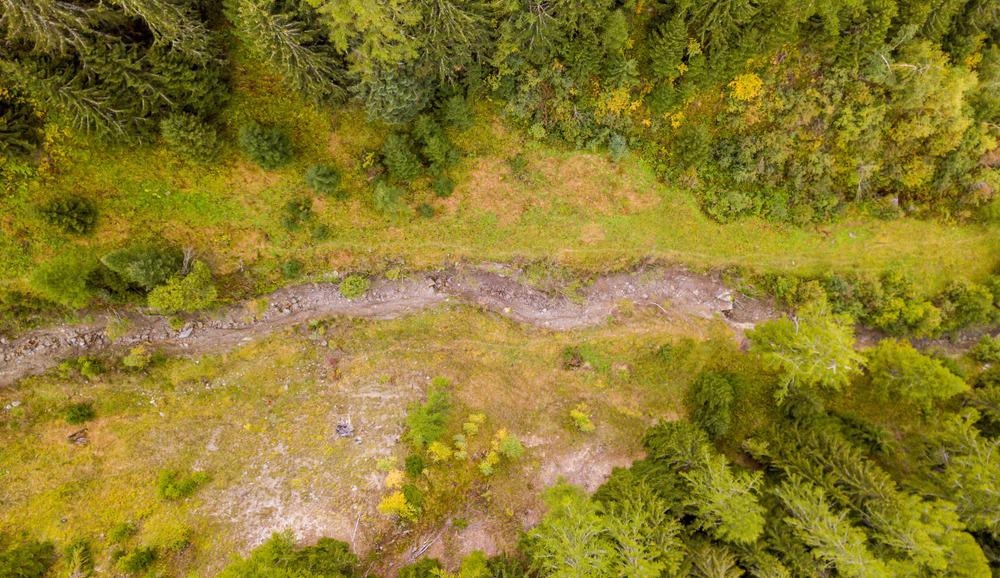
Image Credit: swissdrone/Shutterstock.com
Fast-moving volumes of water laced with soil, fragmented rocks, and even huge boulders racing down the mountainside represent a significant threat to human life, property, and the environment. It is essential to detect these debris flows quickly to prevent loss, but current early warning systems often detect the fast-approaching danger too late.
Conventional instruments - sensors including geophones, radar and laser devices, and video cameras - need to be positioned in easily accessible, low-elevation valley sections. Although reliable, their location in the lower section of the valley restricts warning times to a few minutes.
Researchers at ETH Zurich and the Swiss Federal Institute for Forest, Snow and Landscape Research (WSL) have developed an early warning alarm for debris flow at Illgraben, a steep-sided mountain basin over the Rhone Valley in Switzerland, using seismic monitoring and machine learning (ML).
Their system is a crucial step towards next-generation debris flow alarms. The detector recognizes debris flow much earlier, identifying even the smallest vibration caused shortly after flow initiation from a safe distance. They estimate warnings could be provided up to 20 minutes earlier than current devices.
Illgraben: the Perfect Location
Mudslide and debris flow often occur during heavy rainfall in steep alpine landscapes where they rush through gorges and mountain streams in an uncontrolled, haphazard manner at several meters per second. The number of these destructive avalanches has increased due to climate change, which melts permafrost and causes other extreme weather events, making their occurrence more likely.
Illgraben is one of the most geologically unstable valleys in Switzerland, the perfect natural laboratory for this study. WSL has operated an observatory here in the canton of Valais with measuring stations at Illgraben for more than two decades, allowing them to witness debris flow formation and movement.
For this study, the researchers implemented a network of seismometers – usually used to measure earthquakes - around the Illgraben catchment to record vibrations from debris flows.
This means that debris flows can potentially be detected while they are still in higher-elevation and inaccessible areas."
Fabien Walter, ETH Professor of Glacier Seismology.
The next challenging step was to develop a detector capable of distinguishing between the vibrations caused by debris flow and other ground vibrations in a constant stream of seismic data. The team applied machine learning, utilizing signals from 22 mass movements previously recorded in the area, to train a learning algorithm.
The system was then tested under actual conditions with seismic monitoring data in real-time. In summer 2020, 13 debris flows and smaller flood events occurred at Illgraben, and the team’s AI algorithm reliably detected each one, with no single false alarm generated.
"The algorithm recorded even the first seismic signals of debris flows high up in the catchment," says Walter. This increased the warning time by at least 20 minutes compared with existing detection systems at Illgraben; "This is a huge improvement," Walter adds.
The Future of Debris Flow Detection
The study, published in Geophysical Research Letters, demonstrates how seismic data and ML can detect debris flow at an early stage. However, it is unclear if the detector can identify debris avalanches in other areas. Large data sets are needed to train the algorithm but are often unavailable at other debris flow sites. Researchers hope to further develop the algorithm to work with less or no location-specific data.
The novel detector is the first landmark in a wider collaboration between WSL and Swisscom Broadcast to improve the monitoring of mass movements in Alpine regions. The researchers aim to integrate other relevant data sources such as precipitation data and permafrost measurements to their seismic monitoring using fiber optic cables and the Internet of Things.
References and Further Reading
Keller, M. (2021) AI provides debris flow warnings, ETH Zurich, https://ethz.ch/en/news-and-events/eth-news/news/2021/03/ai-provides-debris-flow-warnings.html. Accessed 9 April 2021.
Chmeil, M. et al (2021) Machine Learning Improves Debris Flow Warning, Geophysical Research Letters, https://doi.org/10.1029/2020GL090874. Accessed 9 April 2021.
My Switzerland Illgraben https://www.myswitzerland.com/en-gb/experiences/illgraben/. Accessed 9 April 2021.
Disclaimer: The views expressed here are those of the author expressed in their private capacity and do not necessarily represent the views of AZoM.com Limited T/A AZoNetwork the owner and operator of this website. This disclaimer forms part of the Terms and conditions of use of this website.