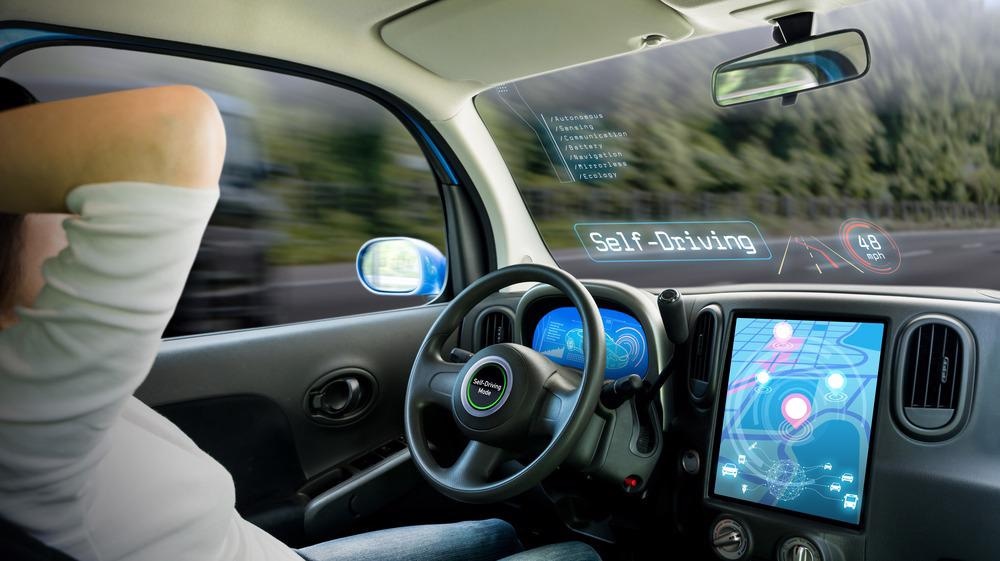
Image Credit: metamorworks/Shutterstock.com
The success or failure of an autonomous vehicle currently depends on how effectively its driving system can handle split-second decisions in hazardous situations. To make driverless cars safer and more commercially viable, the AUTOVISION Spearhead Project from the Europe-based Graphene Flagship consortium is currently creating a new graphene-based, high-resolution image sensor. It will help to quickly identify obstacles and abrupt road changes, even in unusual and challenging driving conditions.
How will the AUTOVISION Spearhead Project Revolutionize Current Self-Driving Technology?
Current self-driving vehicle sensor technology makes use of visible-light cameras to identify obstacles and changes in road conditions. However, these cameras are inadequate in heavy fog. Autonomous cars also employ LiDAR sensors, which involve laser pulses being sent out and reflected back to gauge distances and scan the surrounding area. Unfortunately, LiDAR technology is too slow for use in vehicle accident avoidance.
The Autovision project focuses on developing complementary metal-oxide-semiconductor (CMOS) image sensors that incorporate graphene and quantum dot technology. During the three-year project, research will help improve the sensitivity, pixel size, and functioning speed of a high-resolution image sensor for autonomous vehicles.
The self-driving vehicle sensor technology detects short-wave infra-red radiation (SWIR). Devices that concentrate on this part of the spectrum encounter much less ambient light disturbance and scattering. Because of this, they can produce sharper images. SWIR cameras are customarily made from materials with high costs and a low degree of manufacturability. This negatively affects their use in various markets.
Using CMOS Chips in the Electronics Industry
In the greater electronics industry, CMOS chips are at the center of an ongoing technical revolution. They have made both compact and cost-effective circuits possible, as well as state-of-the-art high-resolution image sensors. However, diversification of the technology has been limited as a CMOS is hard to combine with semiconductors not made of silicon.
Recently, graphene was successfully integrated into a CMOS circuit, making for higher-resolution imaging of visible, UV, and infrared light.
A CMOS sensor's capacity to see within the infrared means that it could easily be incorporated into a driverless car's automatic brake system. This could be used in low light and bad weather conditions. Graphene collision avoidance systems will help to increase the broader acceptance of self-driving vehicle sensor technology.
In 2020, member organizations under the Autovision umbrella announced a technique for the growth and transfer of wafer-scale graphene that uses standard semiconductor equipment. Project members collaborated to outline a suite of camera tests designed to make the Autovision sensor compete with cutting-edge visible cameras, SWIR cameras, and LiDAR systems.
If the Autovision project were to make driverless cars the default type of automobile, it has the potential to prevent as many as 90% of car collisions, which could save countless lives and billions of dollars. Driverless cars are also expected to lower carbon emissions and reduce traffic significantly.
The Growth of CMOS Sensor Technology
CMOS sensors are increasingly finding their way into new, more complex applications, with artificial intelligence expected to take the technology even further.
Electronics manufacturers are implementing and modifying new sensor architecture that increases signal-to-noise ratios, sensitivity, and dynamic range for cameras used in low-light situations. For instance, Sony has decreased the complexity and related build costs in its 5-megapixel 1.75 µm CMOS sensor, which was introduced in 2009.
CMOS performance can be significantly enhanced for high-speed uses by using “global” shutter designs, as opposed to “rolling” shutter designs. While rolling-shutter sensors are cost-effective, they produce unwanted artifacts with fast-moving objects or in moving cameras.
A rolling shutter scans an image vertically, from top to bottom, while a global shutter captures an entire image. As a result, global shutter designs generate more noise and have less dynamic range. These deficits can be reduced but at drastically higher costs. Even so, the low-light and high-speed results of global shutter designs are vastly superior to rolling shutters.
In addition to using global shutter designs, high-resolution image sensors for autonomous vehicles must leverage artificial intelligence to precisely brake and avoid collisions, particularly at high speeds.
CMOS sensor technology can also make for a much broader array of emerging technologies. Machines or robots can use it to distinguish flaws in tiny parts at high speeds for quality assurance, maintenance, or data collection purposes.
With law enforcement departments in large cities already using CMOS sensor technology for surveillance in low-light conditions, the combination of AI and biometric data is anticipated to help stop criminals from getting away with their crimes. First-responder efforts to find missing individuals could save time and lives by using drones to recognize minute signs of human life.
Cutting-edge CMOS sensor technology is already being used in the Mars Perseverance rover mission, which landed on Mars in February 2021. While both CMOS and CCD sensors were used in the mission, the CMOS sensors are expected to be more reliable over time, as CCD sensors are vulnerable to charge transfer degradation.
Resources and Further Reading
Graphene Flagship. Autovision: Graphene collision avoidance systems for autonomous vehicles. [Online] Available at: https://graphene-flagship.eu/innovation/spearheads/c3-sh08-autovision/
Atwell, C. The relentless rise of CMOS image sensors. Fierce Electronics. [Online] Available at: https://www.fierceelectronics.com/sensors/relentless-rise-cmos-image-sensors
Blackman, G. The sensor stories behind the Mars Perseverance images. Imaging & Machine Vision Europe. [Online] Available at: https://www.imveurope.com/analysis-opinion/sensor-stories-behind-mars-perseverance-images
Disclaimer: The views expressed here are those of the author expressed in their private capacity and do not necessarily represent the views of AZoM.com Limited T/A AZoNetwork the owner and operator of this website. This disclaimer forms part of the Terms and conditions of use of this website.