What inspired your research into using smartphone sensors to determine cannabis intoxication in people?
As there are some methods for biological verification of alcohol already in use, such as ankle monitors, we decided to look at whether phone sensor data could be used to detect self-reported heavy drinking (consuming >4/5 drinks per occasion for both women and men, respectively).
We then extended our work with alcohol to our current preliminary study using phone sensors to detect self-reported cannabis intoxication.
Can you provide an overview of how smartphone based features such as GPS and accelerometer can be used to detect intoxication?
When someone is intoxicated from cannabis, they might move more slowly, be less likely to travel long distances and be less likely to show changes in activity, for example, changing from sitting to standing.
The smartphone's accelerometer captures changes in motion and activity that could detect when someone reports being intoxicated. Likewise, the smartphone's GPS sensor captures distance traveled, which could be used to detect reports of cannabis intoxication.
What are some of the current techniques used to detect cannabis intoxication, and what are their limitations?
Existing detection measures, such as blood, urine or saliva tests, have limitations as indicators of cannabis intoxication due to their intrusiveness and inconvenience.
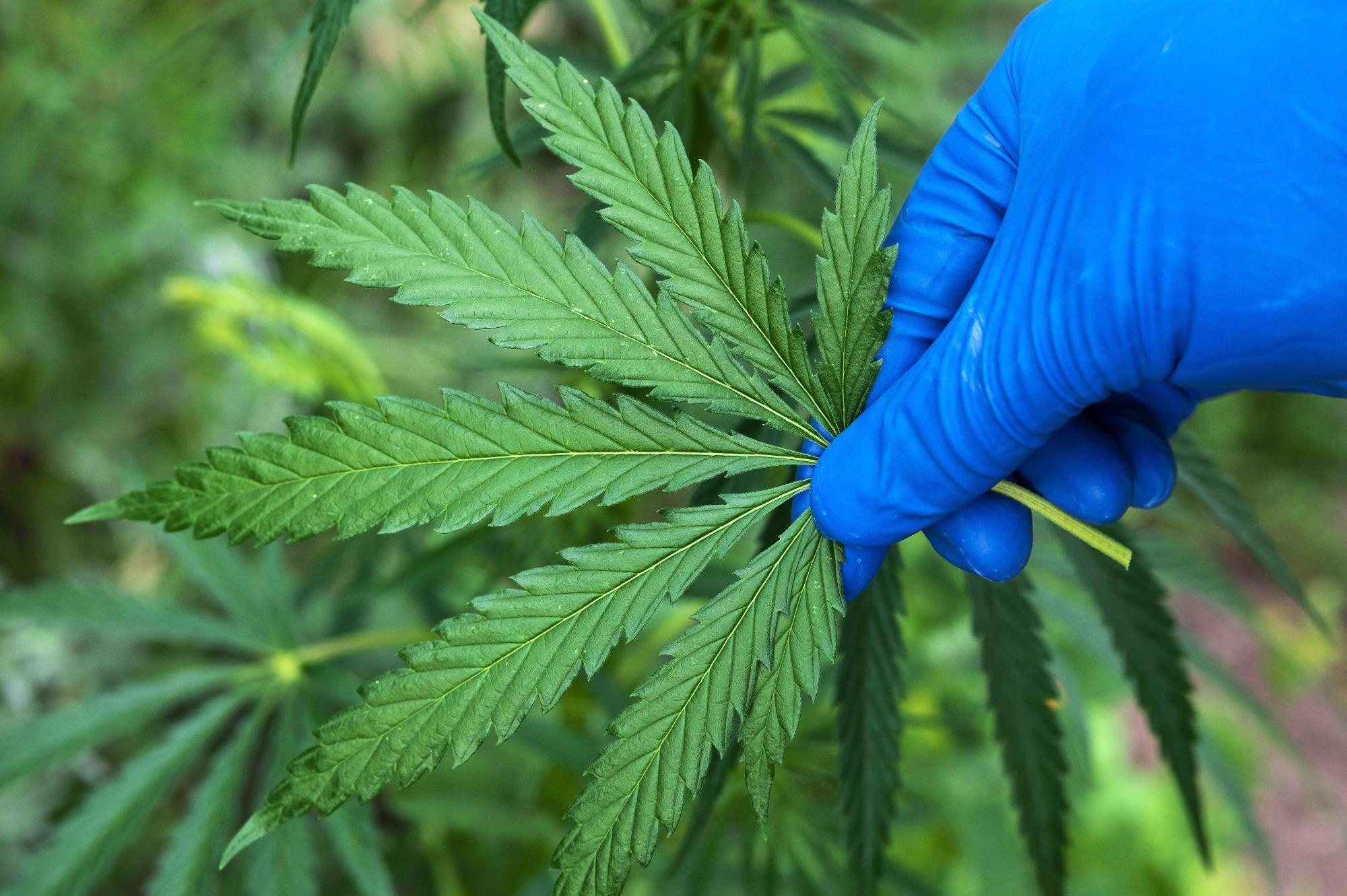
Image Credit: Shutterstock.com/ Andrei Metelev
How can smartphone sensors overcome the challenges faced by conventional detection techniques?
Nowadays, most people have a smartphone. Thus, the method that we tested in this preliminary study of collecting - with consent - and analyzing data from a device that the majority of people already have, is considered a low burden way to detect self-reported cannabis intoxication.
In your research, you mentioned that using smartphone sensors in combination with time features had 90% accuracy in the detection of cannabis intoxication. What are the time features used and why are they so important?
The time features represent the day of the week: weekday vs weekend, and the time of day: morning, afternoon or evening.
Time features can be important as some individuals tend to have routines when it comes to cannabis use. For example, some people may use it on certain days of the week and at specific times of the day. It is, therefore, important to take time features into account.
However, the model using a combination of time features and phone sensor data had the best accuracy in detecting reports of cannabis intoxication.
How will your research also potentially help reduce cannabis-related harm?
The ability to accurately detect when someone is intoxicated from cannabis provides the opportunity to deliver a "just-in-time" notification that can raise a person's awareness of possible impairment associated with cannabis intoxication.
These notifications would be developed in collaboration with participants and provided when and where they might be most useful, to protect personal safety and reduce cannabis-related harm.
What did you discover based on your results and findings?
We found that the combination of time features and phone sensor data had 90% accuracy in detecting self-reported cannabis intoxication. The most important and effective phone sensor features that detected cannabis intoxication came from a GPS (distance traveled) and an accelerometer (activity level).
The GPS indicated a smaller distance traveled, and the accelerometer indicated a smaller number of changes in activity when a participant reported cannabis intoxication.
Did you come across any challenges during your research, and if so, how did you overcome them?
Some challenges involved technological "glitches" (e.g., operating system upgrades) that required our team to be vigilant, rapidly fixing issues as they arose.
We also worked closely with participants to discuss confidentiality, and the study's methods used to protect the confidentiality of the data collected, including the study's National Institutes of Health Certificate of Confidentiality, which protects the confidentiality of research data.
What does the future of smartphone sensors look like to you?
In the future, interest in smartphone sensors as a low burden tool to detect specific behaviors (e.g., intoxication) and to monitor change in certain disease conditions (e.g., Parkinson's Disease) will increase. However, issues such as protecting confidentiality, and replicating results will need to be addressed.
What are the next steps for your research?
An important next step for our research is replicating the study results in a larger, more diverse sample.
This preliminary study did not include any notifications or interventions. In future work, to develop any notification that might be delivered, we would work closely with participants, community members and stakeholders to develop messages that are both timely and relevant.
It would also be useful to include biological verification of self-reported cannabis intoxication in the future.
For more information on Dr. Chung's research, visit this link.
About Dr. Tammy Chung
Dr. Chung is a Professor of Psychiatry and Director in the Center for Population Behavioral Health, Rutgers Institute for Health, Health Care Policy and Aging Research. Dr. Chung’s research focuses on adolescent and young adult substance use: assessment and diagnosis, clinical course and intervention. She graduated from Rutgers (1998 PhD in Clinical Psychology), completed post-docs at Brown University and the University of Pittsburgh and was a Professor at the University of Pittsburgh before joining the Rutgers faculty.
Current projects evaluate the use of mobile technology for substance use assessment and intervention, and examine racial disparities in risk for substance use and sexually transmitted infection/HIV in the Pittsburgh Girls Study (a large longitudinal community sample, N=2450, followed from childhood to young adulthood).
The Center for Population Behavioral Health's (CPBH) mission is to advance theory-driven, interdisciplinary and translational research in four key thematic areas: healthy aging, health equity, health policy, and health technology with the central aim of improving population health.
Disclaimer: The views expressed here are those of the interviewee and do not necessarily represent the views of AZoM.com Limited (T/A) AZoNetwork, the owner and operator of this website. This disclaimer forms part of the Terms and Conditions of use of this website.