Artificial intelligence (AI) or machine learning-based techniques are on the rise in the health sector. Artificial intelligence programs can help make healthcare more customized, predictive, preventive, and interactive, while also enabling healthcare systems to save money.
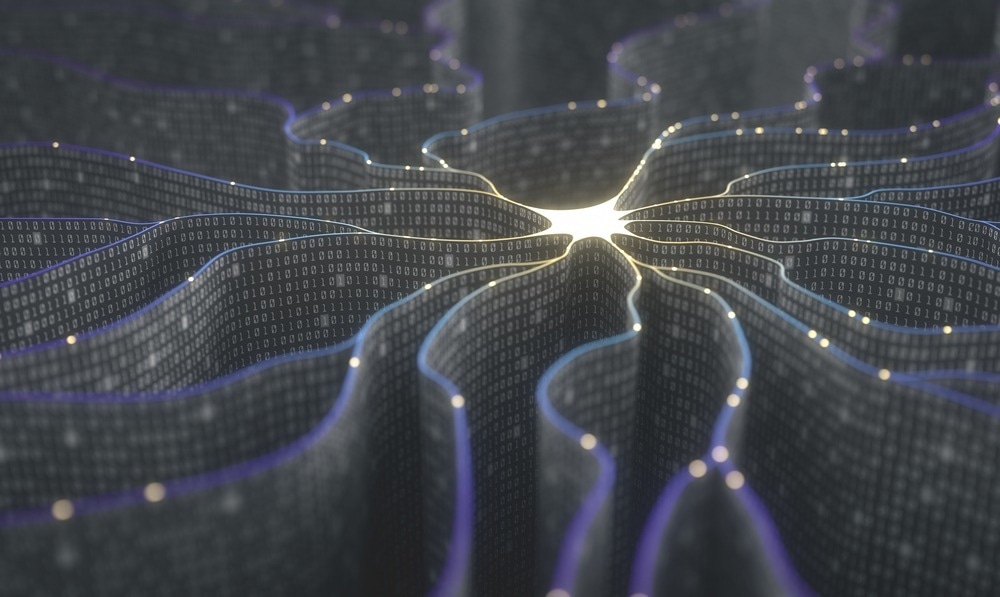
Image Credit: ktsdesign/Shutterstock.com
Machine learning for biomarker identification, industrial robot surgery, medical outcome prediction, monitoring patients, inferring health status from wearable devices, and image-based diagnostics are all popular application fields. Imaging data, electrophysiological data, and genetic data are the most prevalent forms of data explored in AI literature for healthcare.
Algorithmic bias is a serious problem in healthcare and every industry that uses data-driven algorithms. “What humans feel when a machine-learning model gives a systematically erroneous output” is algorithmic bias. Algorithms can be ethically, statistically, or socially prejudiced, and there is plenty of evidence that bias exists in AI.
Sensors may have intrinsic bias and may not operate as effectively for some groups as they do for others. The sensor, which may be included in imaging equipment, a wearable device, or another medical device, generates a measurement, which is then input into an AI or machine-learning-powered algorithm, which produces a result or score that can be used to guide patient treatment.
Figure 1 depicts a flow that is applicable to several AI applications that use sensor data.

Figure 1. Flow of data from sensor to algorithmic output. Image Credit: Bartlett, et al., 2022
Any inaccuracy in sensor measurement, whether due to bias, noise, or another cause, has the potential to input false data into an algorithm, lowering the accuracy of the algorithm’s ultimate output. The influence of sensor precision on sensor or machine-learning-based fall risk assessments is still an open subject in research.
This study published in IPEM-Translation provides a simulation-based technique for assessing the influence of sensor noise on algorithmic output from the ZIBRIO Stability Scale, a medical device used to measure fall risk. While this technique was created for a single application, it may be used for any device that uses sensor-based information to teach a machine learning algorithm.
The ZIBRIO Stability Scale (previously known as the ZIBRIO SmartScale) was created to give a simple way to assess human standing balance as well as postural stability using posturography. The Stability Scale calculates postural stability scores (PS scores) using the Briocore algorithm, which is given as numbers ranging from 1 (worst balance) to 10 Brios (best balance).
The PS score is calculated by the Briocore algorithm using 60 seconds of center-of-pressure (COP) data (3600 samples) recorded during a postural stability test. The Stability Scale’s COP values were determined to be within 0.5 mm of those recorded from a “gold-standard” laboratory force plate in prior research.
The COP data is obtained using four load cell sensors situated on the device platform’s four corners. Figure 2 shows the device in action.
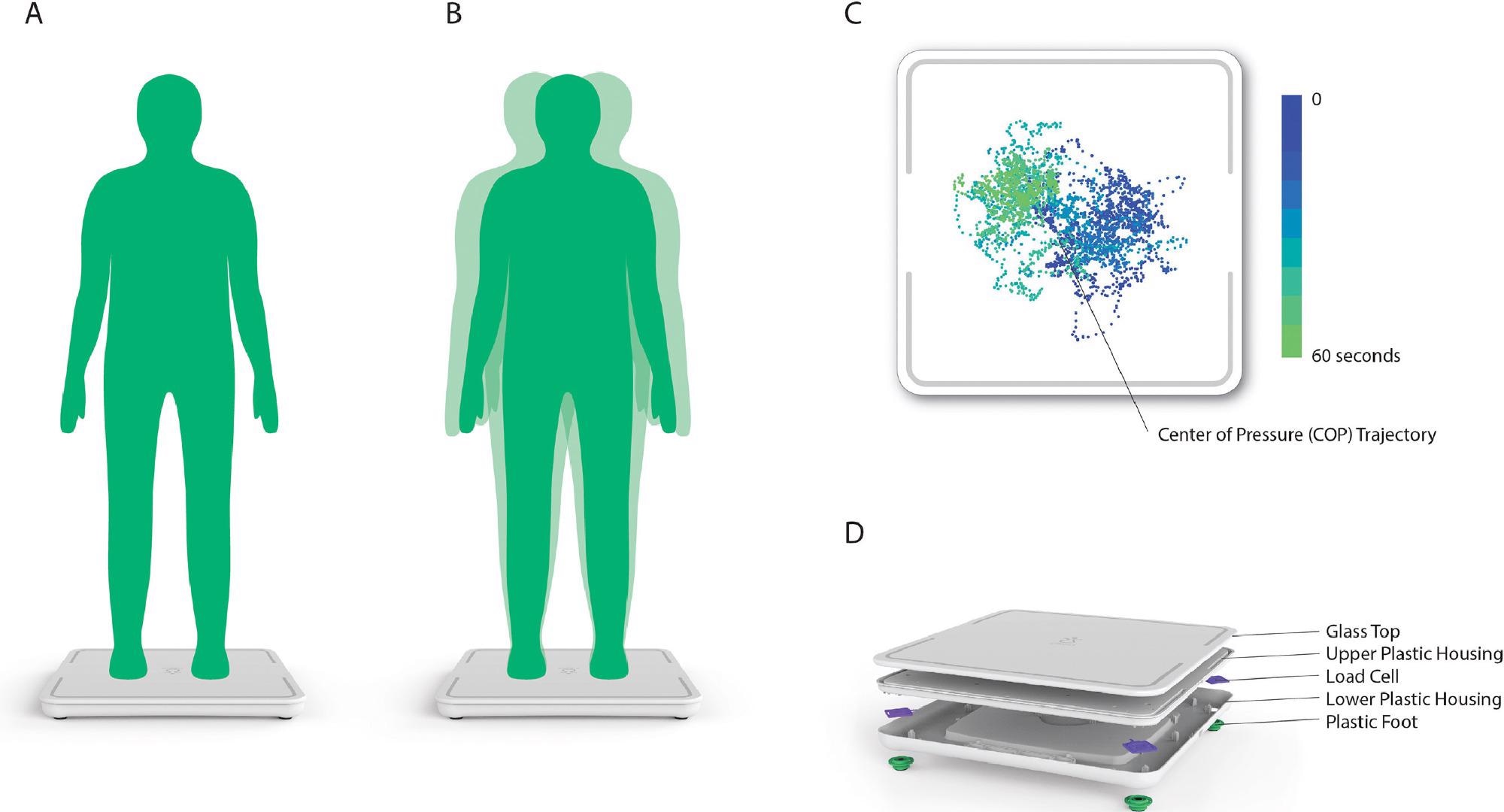
Figure 2. Reproduced (with permission) from the work by Forth et al. A) Person stands still on device for 60 s for postural stability assessment. B) During the assessment, the person’s body will make small movements, which are captured in COP measurements. C) Plot of COP measurements collected by the scale during the postural stability assessment. D) Location of load cell sensors inside the device. Image Credit: Bartlett, et al., 2022
Methodology
The perturbations were carried out in four distinct ways: just COPx (Condition 1), just COPy (Condition 2), just COPx and COPy by the same amount (Condition 3), and just COPx and COPy by different quantities (Condition 4). Figure 3 depicts the situation graphically.
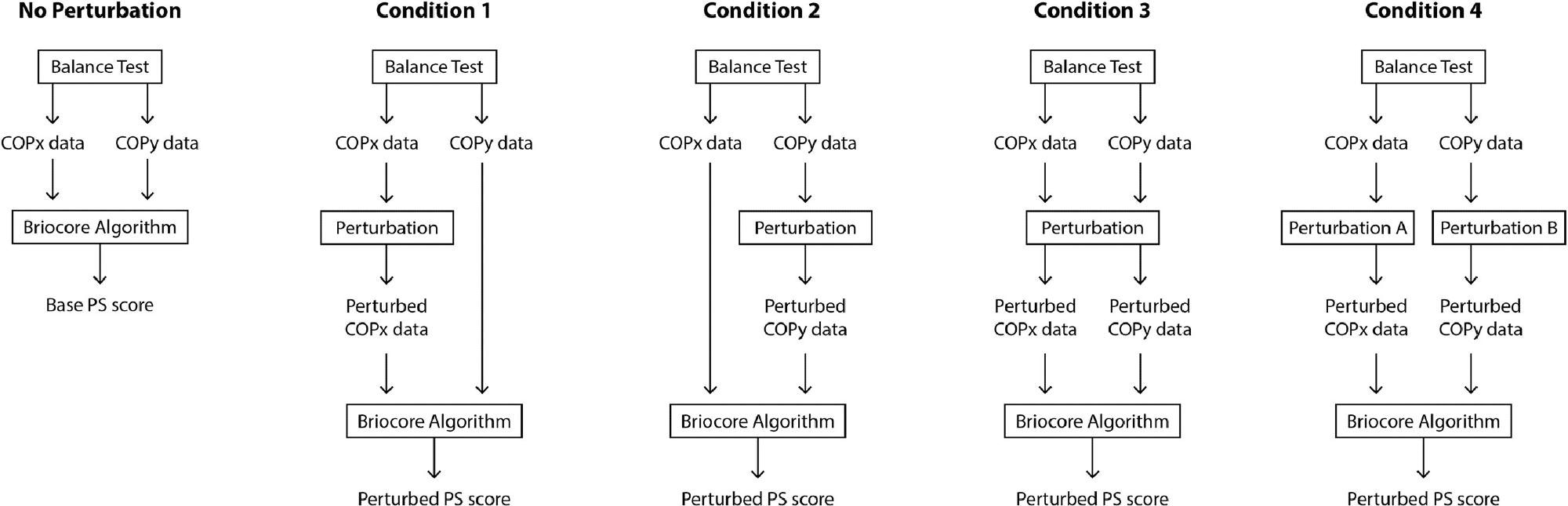
Figure 3. Visualization of PS score perturbation process compared to base PS score calculation process (“No Perturbation” condition). Image Credit: Bartlett, et al., 2022
Results
In the COP measurements, a perturbation magnitude of +/- 3 mm was used as the greatest acceptable error level. The mean agreement between the basic PS score and the disturbed PS score was 79.01+/-17% at +/- 3 mm of inaccuracy in Figure 4.
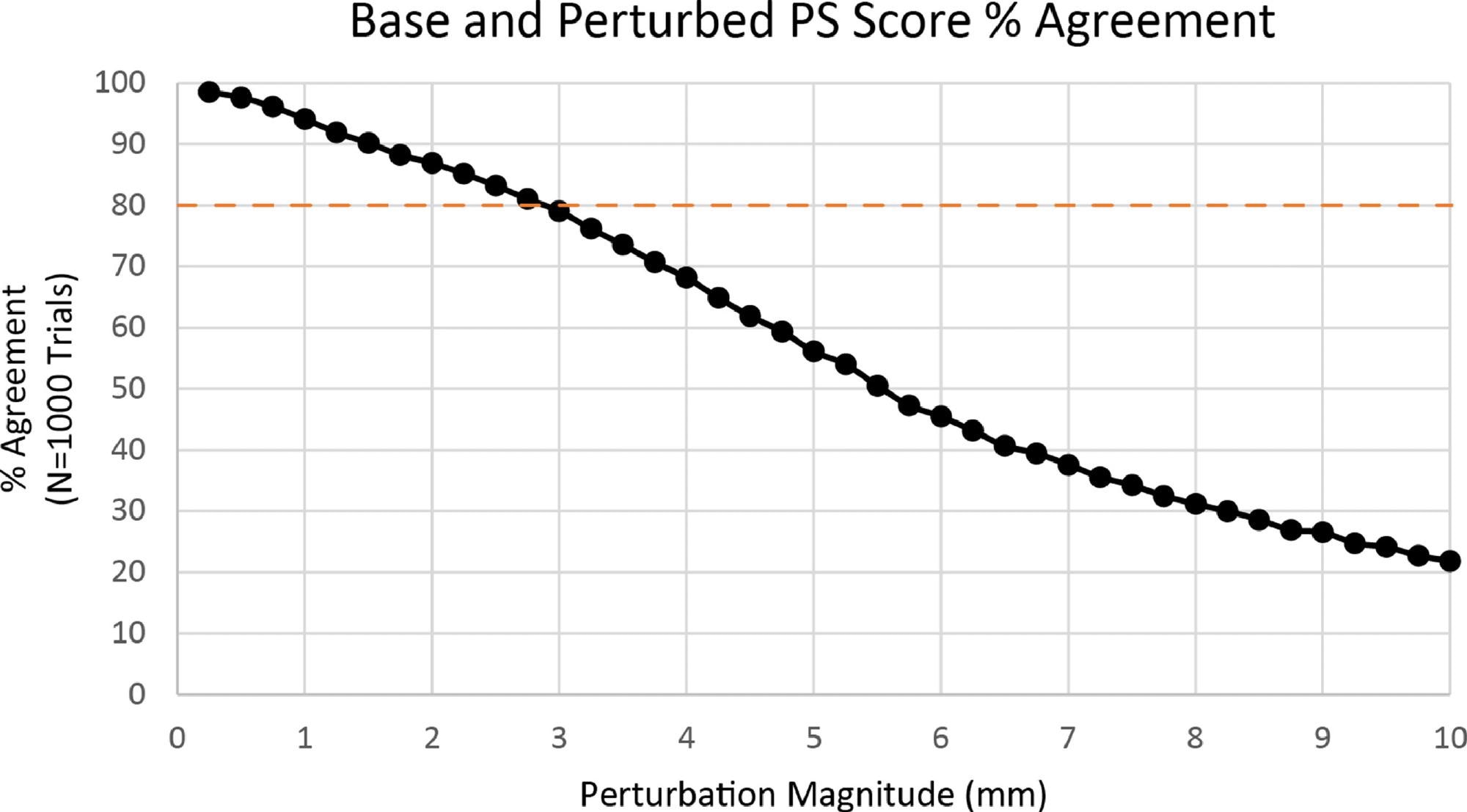
Figure 4. Percent agreement between base PS score and perturbed PS score. Image Credit: Bartlett, et al., 2022
The percentage of perturbed PS scores equal to or less than the basic PS score was 95.54+/8% at +/- 3 mm shown in Figure 5.
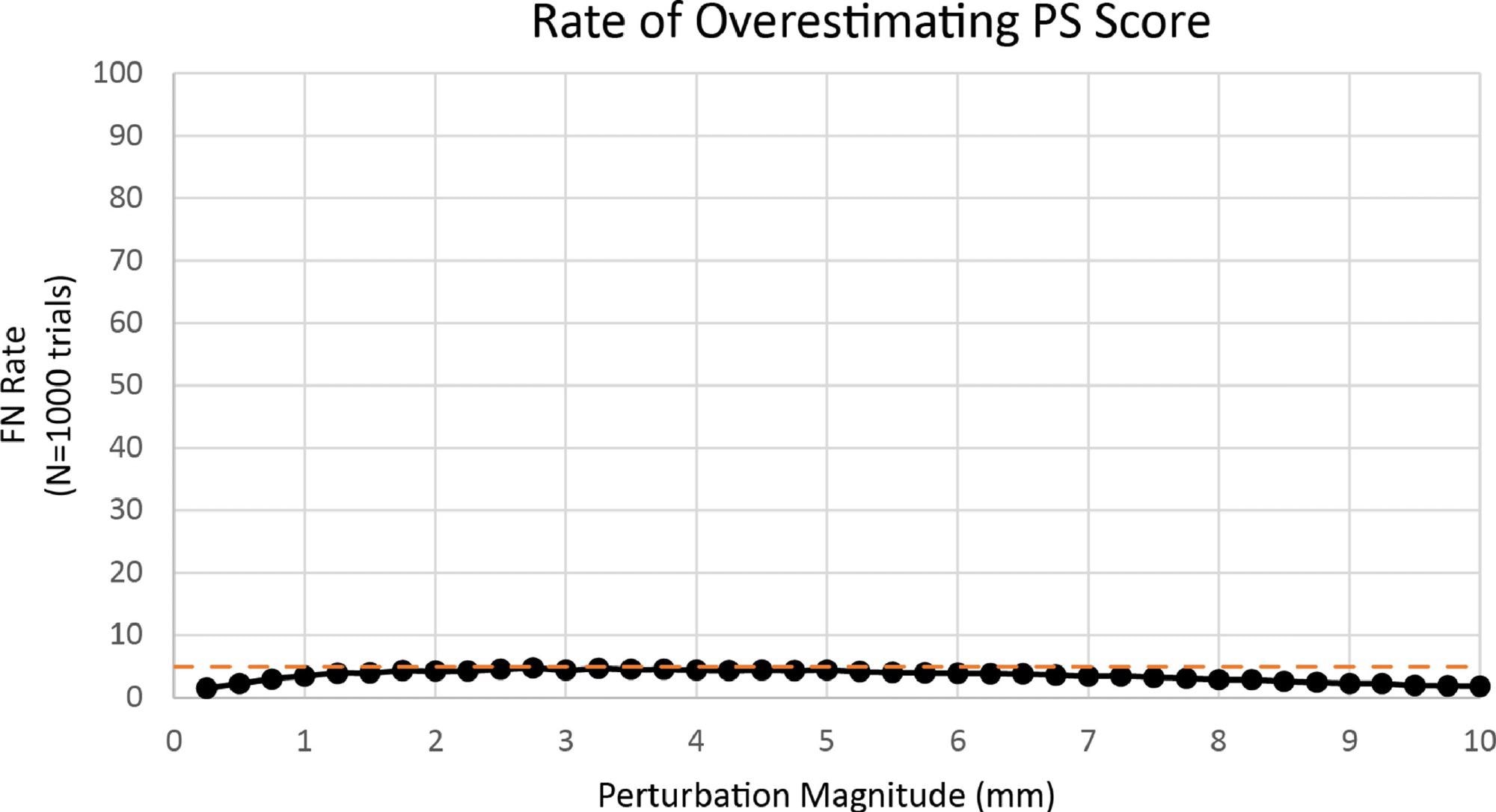
Figure 5. Rate of overestimating PS score. Image Credit: Bartlett, et al., 2022
Two further datasets were added to test the sensitivity of a PS score of “2 Brios” following one of the three randomly chosen datasets.
The unrounded PS score in dataset 2C equaled 2.4666, indicating that it was a one-of-a-kind example that was very sensitive to altering the rounding direction if the score rose by as little as 0.0334 points.
Discussion
Given the growing number of medical devices that give algorithm-based scores, it is critical for device makers to guarantee that the sensors used in these devices have a sufficient resolution so that the algorithm’s outputs are accurate.
The influence of sensor accuracy on the final score output is not as obvious when sensor readings are utilized as input for an algorithmic score. To solve this issue, researchers designed a mechanism for simulating sensor noise at various levels and evaluating how this might affect the algorithmic score output.
Based on the findings of experimental calculations, the tolerable error for COP readings in the ZIBRIO Stability Scale device was set at +/- 3 mm. At this amount of noise, over 80% of the perturbed PS scores (79.01%) were comparable to the basic PS value; hence +/- 3 mm was considered to be an acceptable condition.
Engineers believe that +/- 3 mm precision can be achieved using compact, lightweight, low-cost sensors, which might be employed to manufacture very small, portable posturography devices.
When compared to a gold standard laboratory force plate, both the current and earlier generation of Stability Scale prototypes had maximum absolute errors below the required +/- 3 mm, and can be considered adequate in determining COP to evaluate postural stability using the Briocore algorithm.
When measuring a 65 kg load (637.43 N), 3 mm of inaccuracy in the x or y direction equals 1.09 kg of measurement error across all four sensors, or 0.27 kg of evaluation error in a single sensor, according to the COP equation.
Despite the fact that the findings imply that the sensors are in the fourth generation, only 32 postural stability assessments were used in the study. During algorithm sensitivity evaluations, simulations were done to account for unforeseen variations in COP measurement accuracy, which might be caused by mechanical difficulties, electrical noise, or sensor faults or damage.
Conclusion
Sensor accuracy standards are not always clear when medical sensors are utilized to create algorithmic scores or outputs. When utilized in real-world situations, sensors are unlikely to deliver precise readings all of the time; hence, device makers must establish acceptable sensor accuracy criteria.
In this study, researchers present a simulation-based technique for determining such accuracy criteria and their influence on algorithmic score outputs. While researchers have given a reliable approach for determining how sensor inaccuracy affects algorithmic score outputs, sensor accuracy tolerance judgments in various applications may differ.
Manufacturers of medical devices must define sensor accuracy standards based on clinical and ethical implications, giving particular attention to the impact of inaccurate predictions on patient care. For the future acceptance of algorithm-derived healthcare insights, clear assessments of algorithm noise susceptibility will be required.
Journal Reference:
Bartlett, K.A., Forth, K.E. and Madansingh, S.I. (2022) Characterizing sensor accuracy requirements in an artificial intelligence-enabled medical device. IPEM-Translation, p.100004. Available Online: https://www.sciencedirect.com/science/article/pii/S2667258822000024.
References and Further Reading
- Bhardwaj, R., et al. (2017) A Study of Machine Learning in Healthcare. In: 2017 IEEE 41st Annual Computer Software and Applications Conference (COMPSAC), Turin: IEEE. doi.org/10.1109/COMPSAC.2017.164.
- Jiang, F., et al. (2017) Artificial intelligence in healthcare: past, present and future. Stroke and Vascular Neurology, 2(4), pp. 230–243. doi.org/10.1136/svn-2017-000101.
- Rong, G., et al. (2020) Artificial Intelligence in Healthcare: Review and Prediction Case Studies. Engineering, 6(3), pp. 291–301. doi.org/10.1016/j.eng.2019.08.015.
- Bohr, A & Memarzadeh, K (2020) Chapter 2 - The rise of artificial intelligence in healthcare applications. Artificial Intelligence in Healthcare, pp. 25–60. doi.org/10.1016/B978-0-12-818438-7.00002-2.
- Yu, K-H., et al. (2018) Artificial intelligence in healthcare. Nature Biomedical Engineering, 2, pp. 719–731. doi.org/10.1038/s41551-018-0305-z.
- Char, D. S., et al. (2020) Identifying Ethical Considerations for Machine Learning Healthcare Applications. The American Journal of Bioethics 20(11), pp. 7–17. doi.org/10.1080/15265161.2020.1819469.
- Chen, I. Y., et al. (2021) Ethical Machine Learning in Healthcare. Annual Review of Biomedical Data Science, 4, pp. 123–144. doi.org/10.1146/annurev-biodatasci-092820-114757.
- Williams, R. M., et al. (2022) Not Another Troller! On the Need for a Co-Liberative Consciousness in CS Pedagogy. IEEE Transactions on Technology and Society, 3(1), pp. 67–74. doi.org/10.1109/TTS.2021.3084913.
- Fazelpour, S & Danks, D (2021) Algorithmic bias: Senses, sources, solutions. Philosophy Compass, 16(8), p. e12760. doi.org/10.1111/phc3.12760.
- Nelson, G S (2019) Bias in Artificial Intelligence. North Carolina Medical Journal, 80(4), pp. 220–222. doi.org/10.18043/ncm.80.4.220.
- Koene, A., et al. (2018) IEEE P7003TM Standard for Algorithmic Bias Considerations. In: ACM/IEEE International Workshop on Software Fairness, pp. 38–41. doi.org/10.1145/3194770.3194773.
- Paulus, J K & Kent, D M (2020) Predictably unequal: understanding and addressing concerns that algorithmic clinical prediction may increase health disparities. npj Digital Medicine, 3, p. 99. doi.org/10.1038/s41746-020-0304-9.
- Sjoding, M. W., et al. (2020) Racial Bias in Pulse Oximetry Measurement. The New England Journal of Medicine, 383, pp. 2477–2478. doi/full/10.1056/nejmc2029240.
- Ferreira, R. N., et al. (2017) Fall Risk Assessment Using Wearable Sensors: A Narrative Review. Sensors, 22(3), p. 984. doi.org/10.3390/s22030984.
- Usmani, S., et al. (2017) Latest Research Trends in Fall Detection and Prevention Using Machine Learning: A Systematic Review. Sensors, 21(15), p. 5134. doi.org/10.3390/s21155134.
- Arachchige, K., et al. (2017) Occupational Falls: Interventions for Fall Detection, Prevention and Safety Promotion. Theoretical Issues in Ergonomics Science, 22(5), pp. 603–618. doi.org/10.1080/1463922X.2020.1836528.
- Bartlett, K. A., et al. (2017) Validating a low-cost, consumer force-measuring platform as an accessible alternative for measuring postural sway. Journal of Biomechanics, 90, pp. 138–142. doi.org/10.1016/j.jbiomech.2019.04.039..
- Forth, K. E., et al. (2017) A Postural Assessment Utilizing Machine Learning Prospectively Identifies Older Adults at a High Risk of Falling. Frontiers in Medicine, 7, p. 591517. doi.org/10.3389/fmed.2020.591517.