Several years ago, a new direct method was developed for detecting the resin flow front during the Liquid Resin Infusion process in an industrial setting. The reliability of resin infusion data has been improved by using a sensor system in resin transfer molding (RTM).
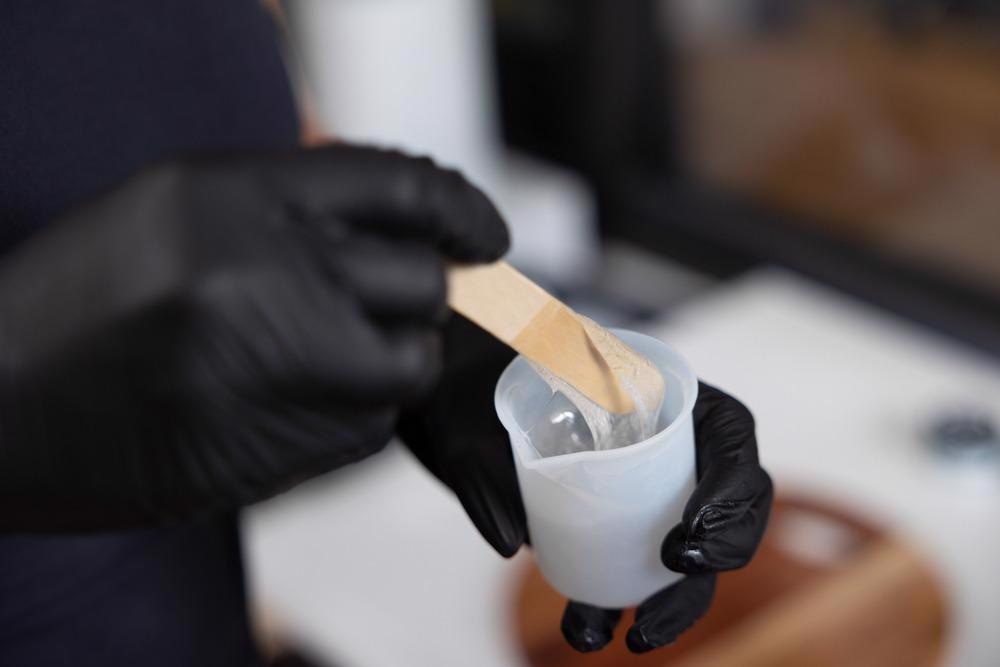
Image Credit: Eric fdo/Shutterstock.com
Adopting this technique is critical to decrease the number of initial experimental attempts and promote a "right-first-time" approach and, therefore, the time and money spent producing components.
Global business has shifted its focus to environmental sustainability, necessitating decreased waste and resource consumption (e.g., energy, water, and materials). Consequently, production needs to become more efficient and intelligent.
Resin Transfer Molding
Manufacturing continuous fiber-reinforced thermoset composites using resin transfer molding (RTM) is a relatively new process. The procedure involves three phases: first, the substance is placed into a mold cavity with a dry fiber reinforcement (pre-form). Forced resin impregnation is then carried out (filling stage). Finally, the mold is heated, the thermosetting matrix material is cross-linked, and the composite cures.
Data Collection by Sensors in Resin Processes
One of the first stages toward intelligent manufacturing is defining the measures needed to improve part quality and output and the sensors required to acquire those measurements. Microwave, ultrasonic and electromagnetic waves can be used to detect dielectric, thermal flux, fiber optic, and other non-contact wave sensors.
Reasons to Use Sensors in the Resin Industry
Sensor networks that can monitor and regulate the manufacturing process in-situ, depending on several quantities (such as pressure and temperature) and the status of the material, are becoming increasingly common in the parts industry. (e.g., viscosity, polymerization, crystallization).
Since mixing, infusion, cure, and polymerization can now be seen as complete, cycle times may be slashed. To validate qualities, sensors can also eliminate the need for post-processing inspection and testing, saving the industry a lot of money.
To better understand and visualize the process, a sensor may be useful. Using Cosimo’s sensor network, the thermoplastic RTM process and material behavior may be seen in complete detail. The sensor at each point is used to obtain information such as local changes at the different thicknesses of a component or in materials (as in foam core), the overall shape of the resin flow, and the time it takes the resin to permeate the preform. The sensor also provides information regarding the degree of polymerization.
Using them can shorten the time it takes for the procedure to complete. Two-part snap-cure epoxy may be tested for homogeneity at any spot in the mold where sensors have been put, and this can be done throughout the RTM process. Faster cure resins, such as those used in urban air mobility (UAM), might benefit since they would have shorter cure times than existing one-component epoxies like RTM6. Monitoring resin flow and curing with sensors is common in composites, allowing these processes to be terminated when they are complete rather than using cautious time-based recipes that include additional time for curing.
A goal for composite manufacturing is to have automated closed-loop control during the manufacturing process. Digital model sensors and algorithms are now being used in industries to simulate the processes and forecast results for the completed performance of the part.
These processes include the fiber spray preforming (with the snap-cure epoxy) for RTM, placement of prepreg tape (automated), and high-pressure T-RTM for PA6 composite. For example, temperature and pressure may be increased or decreased in real-time, resin intakes can be closed when the infusion is complete, or a heated mold or press can be opened after the goal cure has been achieved with closed-loop control.
Dielectric Sensor by the National Composite Center (NCC)
New dielectric sensor technology has been developed by the National Composites Centre (NCC) in the United Kingdom (UK) to collect data from liquid resin operations. The NCC conducted testing of NCC’s 7 Bar and 180°C test conditions for capacitance sensors that assess permittivity in a surrounding medium.
The sensors, according to the center, were able to accurately identify resin as it flowed within the preform and as it cured. The sensors had no negative impact on the process or the component's quality. AMRC in Sheffield and Meggitt collaborated with the team to incorporate the sensors inside a closed resin transfer molding (RTM) tool, requiring no alterations to the machine itself. This sensor has successfully collected resin flow and cure degree data in experiments, aligning with prior reports and theoretical models' results.
Intended for a civil aviation jet engine air-oil heat exchanger on a large-scale composite housing part, the sensors proved to be sturdy and effective.
Future Developments
In the last several years, RTM's filling stage has been widely explored. Based on Darcy's law and the mechanics of resin impregnation, flow models have been constructed to predict the filling behavior of containers. Through these studies, it has been able to better predict fill times, assess the likelihood of dry spots forming, and investigate the impact of various process variables on those times. NDT (non-destructive testing) inspections of future components are to be minimized as we go towards digital manufacturing.
References and Further Reading
GARDINER, G. (2022, 03 01). Sensors: Data for next-gen composites manufacturing. Retrieved from Composites World: https://www.compositesworld.com/articles/sensors-data-for-next-gen-composites-manufacturing
NEHLS, G. (2022, 5 1). NCC sensor technology unlocks digital potential for liquid resin processes. Retrieved from Composite World: https://www.compositesworld.com/news/ncc-sensor-technology-unlocks-digital-potential-for-liquid-resin-processes
Skordos, A. A. (2000). A dielectric sensor for measuring flow in resin transfer molding. Measurement Science and Technology. https://doi.org/10.1088/0957-0233/11/1/304
Wang, P. (2011). Monitoring the resin infusion manufacturing process in an industrial environment using distributed sensors. Journal of Composite Materials. https://doi.org/10.1177/0021998311410479
Disclaimer: The views expressed here are those of the author expressed in their private capacity and do not necessarily represent the views of AZoM.com Limited T/A AZoNetwork the owner and operator of this website. This disclaimer forms part of the Terms and conditions of use of this website.