The maturation of artificial intelligence (AI) technologies like machine learning and artificial neural networks, combined with a drastic rise in available computer processing power, has had a significant impact on the sensors industry. This article explores some recent examples of AI being used in the sensors industry.
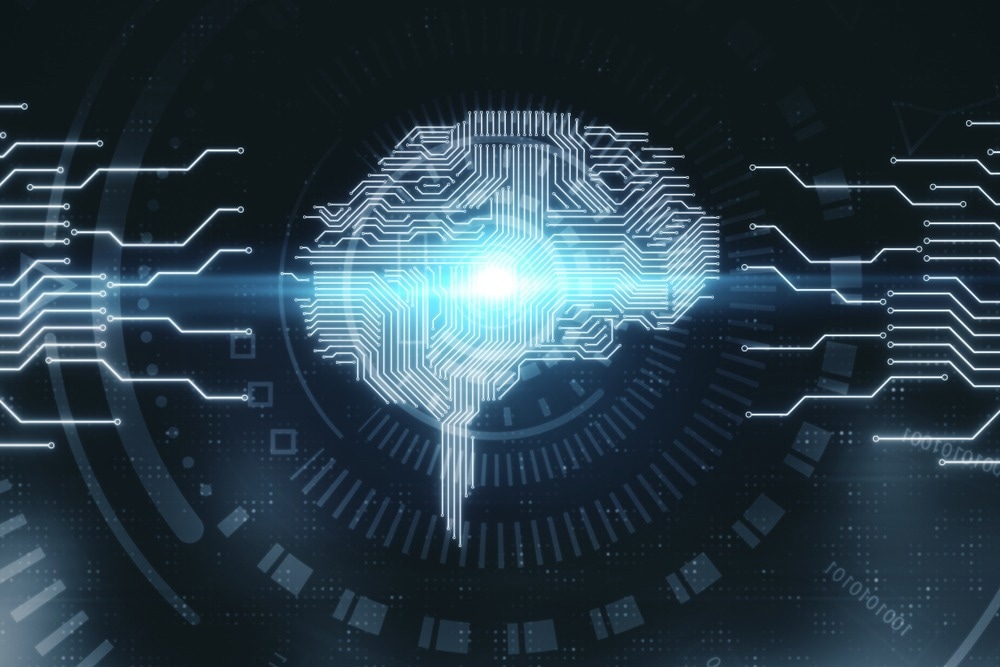
Image Credit: Peshkova/Shutterstock.com
Intelligent Sensing
Intelligent sensors are detection devices with built-in computational power. They can test and validate their own sensing results, as well as adapt parameters and identify new sensing targets without human intervention.
Intelligent sensor systems may also execute self-directed outputs, often via other devices linked up with the sensor or sensors in an Internet of Things (IoT) network. For example, an intelligent detection device may be used to control building temperature automatically, opening windows or turning on heating systems as the building naturally changes temperature.
Intelligent sensors require bespoke hardware to work, known as signal conditioning circuitry. This monitors the sensor and controls it, as well as sends output control signals to other connected devices.
Intelligent detection devices are reportedly more accurate than traditional counterparts due to their capacity for self-calibration and self-diagnosis of faults in the sensor system.
Machine Learning and Inverse Design for Sensor Development
As well as imbuing sensors and detection devices with artificial intelligence, the sensors industry also uses cutting-edge AI methods to develop sensing platforms.
A study by University of California, Los Angeles (UCLA) engineers shows how inverse design and machine learning approaches can be used in sensor development. The article, published in Nature Machine Intelligence in 2021, argues that detection devices could be fundamentally redesigned using these AI methods.
Inverse design is a design approach that has recently become popular in natural sciences and engineering alike. In an inverse design method, the configuration of the end result is determined from targeted searches, which are performed automatically by computers. Here, computers are given an optimization problem, in which they are instructed to keep iterating new versions of the design until certain set parameters are met.
Machine learning is an AI approach that instructs computers to process data in such a way as to learn from the data and use that learning to determine future behaviors, including what else to look for in the data set.
The study shows how using these AI methods to iteratively analyze sensing outcomes can result in low-cost, compact, or more accurate sensor systems. The authors predict that these methods will introduce a new generation of application-targeted sensing implementations, which may be highly non-intuitive and unique.
Embedding Sensors with AI
Microcontrollers are found in numerous electronic devices today, including sensors such as blood pressure monitors and fitness wearables. Recently, Fraunhofer Institute researchers developed an AI system specifically for microcontrollers.
Currently, there are several software applications for machine learning, but these tend to only be available for computers and are based on the Python programming language. This means that neural networks - a form of AI which processes data in a networked fashion that is analogous to the way that brains work - cannot be processed inside microcontrollers.
The Fraunhofer Institute researchers overcame this problem by programming a machine learning library in the C programming language and ensuring it would run on microcontrollers. The library is platform-agnostic, however, so it can also run on Raspberry Pis and popular operating systems.
The team reduced source code to the bare minimum so that the neural network could be trained directly on the sensor itself without resorting to connections with a more powerful computer processor. The platform-agnostic feature of this code also means that neural networks trained on a microcontroller or detection device can be easily ported to a computer and vice versa.
Biosensing Platform with In-Sensor Machine Learning
Another example of detection devices embedding AI functions rather than connecting to a more powerful computer for AI was recently proposed in a 2020 Nature Electronics article by researchers from University of California (UC) Berkeley, University of Bologna (Italy), and IBM Research Zurich (Switzerland).
Wearable devices for monitoring muscle activity based on electromyography on the skin’s surface using machine learning models to classify gestures, which are movements of the body or hand that tell a machine what to do. However, devices that can process information locally cannot typically also handle training and updating the machine learning approach that was used. This results in suboptimal sensing performance in real-life settings.
The researchers developed a wearable surface electromyography biosensing system based on an electrode array that provides adaptive learning capability in the sensor itself. Their results showed high accuracy (over 90%) for gesture recognition.
Strain Sensors with Machine Learning
Engineers from Singapore’s National University of Singapore and Singapore University of Technology and Design, China’s Southern University of Science and Technology and Tsinghua University, and the USA’s University of Maryland published a paper that lays out a machine learning approach to optimize fabrication methods for strain sensors. Strain sensors are important for the emerging class of soft machines.
The team used a support-vector machine classifier that they trained on hundreds of different nanomaterials’ compositions. These went through a dozen active learning loops to fabricate 125 strain sensors.
The method resulted in improved fabrication methods for strain sensors, which the team tested in real-life soft machine applications.
References and Further Reading
Ballard, Z., et al (2021). Machine learning and computation-enabled intelligent sensor design. Nature Machine Intelligence. doi.org/10.1038/s42256-021-00360-9.
Intelligent Sensor. [Online] Techopedia. Available at: https://www.techopedia.com/definition/31462/intelligent-sensor
Machine learning for sensors. (2019) [Online] Phys.org. Available at: https://phys.org/news/2019-06-machine-sensors.html
Moin, A., et al (2020). A wearable biosensing system with in-sensor adaptive machine learning for hand gesture recognition. Nature Electronics. doi.org/10.1038/s41928-020-00510-8.
Morris, A.S., and R. Langari (2021). Intelligent sensors. Measurement and Instrumentation. doi.org/10.1016/B978-0-12-817141-7.00011-6.
Yang, H., et al (2022). Automatic strain sensor design via active learning and data augmentation for soft machines. Nature Machine Intelligence. doi.org/10.1038/s42256-021-00434-8.
Disclaimer: The views expressed here are those of the author expressed in their private capacity and do not necessarily represent the views of AZoM.com Limited T/A AZoNetwork the owner and operator of this website. This disclaimer forms part of the Terms and conditions of use of this website.